MetConSIN
Los Alamos National Laboratory
Description
Microbial communities assemble through a complex set of interactions between microbes and their environment, and the resulting metabolic impact on the host ecosystem can be profound. Microbial activity is known to impact human health, plant growth, water quality, and soil carbon storage which has lead to the development of many approaches and products meant to manipulate the microbiome. In order to understand, predict, and improve microbial community engineering, genome-scale modeling techniques have been developed to translate genomic data into inferred microbial dynamics. However, these techniques rely heavily on simulation to draw conclusions which may vary with unknown parameters or initial conditions, rather than more robust qualitative analysis. To better understand microbial community dynamics using genome-scale modeling, we provide a tool to investigate the network of interactions between microbes and environmental metabolites over time.
Using our previously developed algorithm for simulating microbial communities from genome-scale metabolic models (GSMs), we infer the set of microbe-metabolite interactions within a microbial community in a particular environment. Because these interactions depend on the available environmental metabolites, we refer to the networks that we infer as metabolically contextualized, and so name our tool MetConSIN: METabolically CONtextualized Species Interaction Networks.
Unique Aspects
Novel algorithm for inferring interaction networks from genome-scale models that accounts for the dynamic nature of the community and environment.
Availability
Open source at github.com/LANL
Benefit
Functional model of microbial community metabolism that allows hypothesis generation and testing in-silico for microbiome manipulation and design.
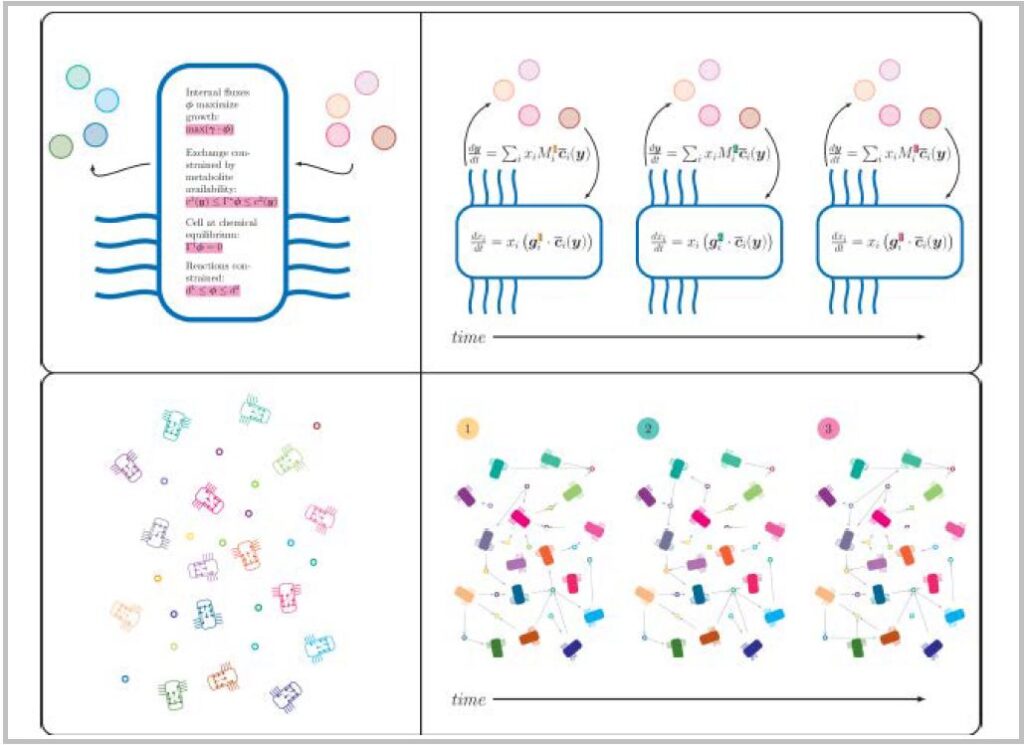
Capability Expert(s)
James Brunner
References
Brunner JD, Gallegos-Graves LA, Kroeger ME (2023) Inferring microbial interactions with their environment from genomic and metagenomic data. PLOS Computational Biology 19(11): e1011661. https://doi.org/10.1371/journal.pcbi.1011661